Data Science Career Paths: Industry, Academia, and Entrepreneurship
Overview
Data science provides a variety of fascinating employment opportunities in business, academia, and other fields. Data scientists use their expertise to tackle practical issues in business, using data to inform choices and create novel solutions. They work in interdisciplinary teams, examine huge datasets, create prediction models, and share findings with stakeholders.
Data scientists participate in cutting-edge research at universities, publish articles, and instruct the next generation. They investigate novel approaches, work with scholars, and expand the amount of information in the area. Additionally, there are prospects in academia for multidisciplinary partnerships, mentoring, and teaching.
Data scientists who are entrepreneurs can create their own companies and goods. They locate market possibilities, create teams, create data-driven solutions, and set up data infrastructures. Entrepreneurs make use of their abilities to develop novel goods, obtain finance, and move through the startup ecosystem. They create relationships, respond to market changes, and promote expansion.
Data scientists require excellent abilities in statistics, programming, machine learning, and domain expertise, which are essential components of a comprehensive Data Science Course. They must also embrace continuous learning, stay updated with tools and technology, and possess strong communication skills. Data science offers significant opportunities to make an impact, drive innovation, and contribute to the rapidly evolving field of data science, whether in business, academia, or other sectors.
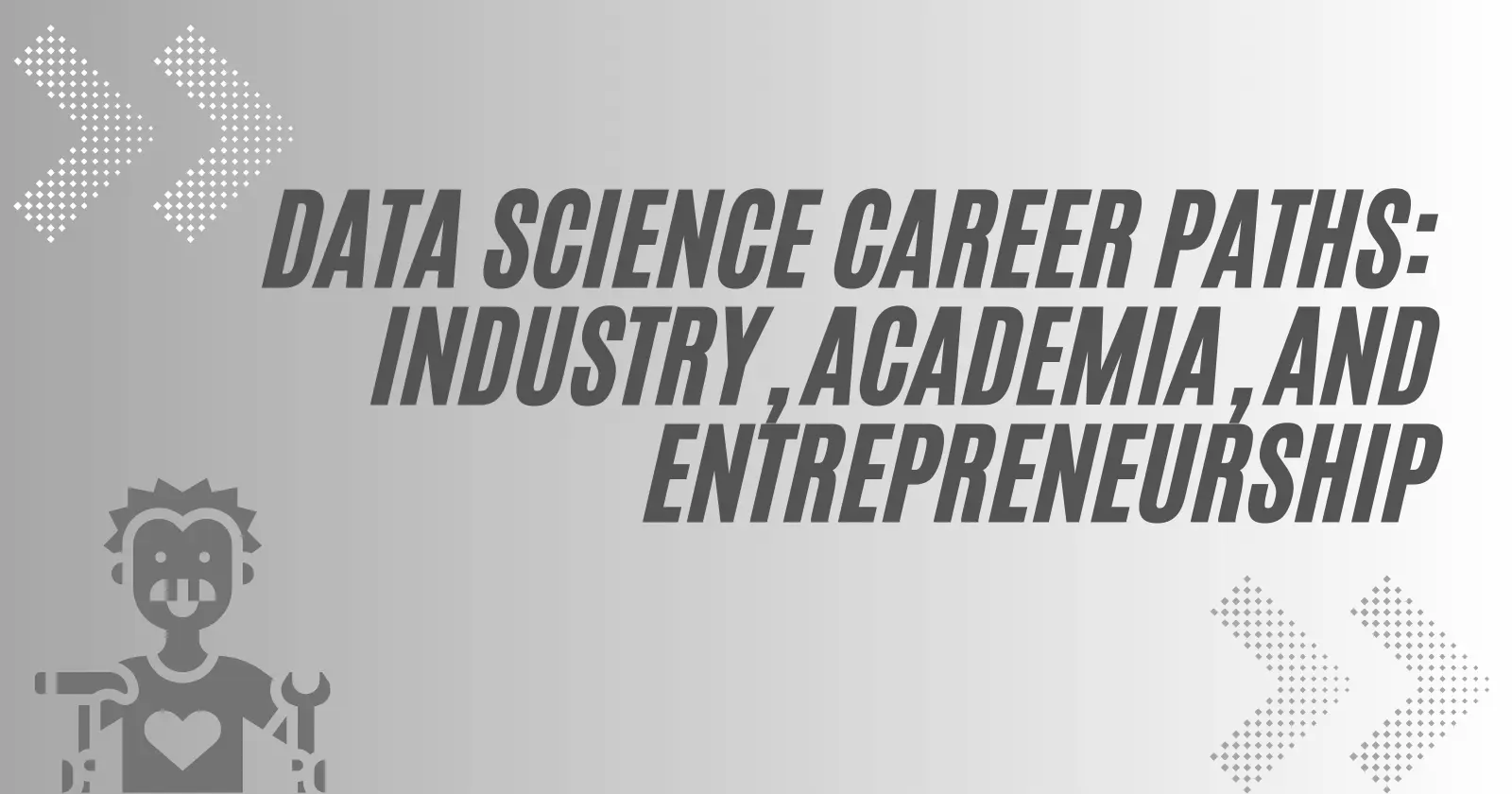
Data Science Career Paths
The recognition of data science as the "hottest job of the 21st century" is indeed a factor that has attracted many individuals to pursue a career in this field. The increasing demand for data scientists in businesses of all sizes is a testament to the growing importance of data-driven decision-making.
One of the compelling aspects of data science is the interdisciplinary nature of the field. Data scientists need to possess a strong foundation in statistics, mathematics, and technologies such as programming and machine learning. This diversity of skills is an asset because it allows data scientists to approach problems from various angles and leverage different techniques to gain insights from data.
The ideal degree to become a data scientist in 2020 is... Data Science and Analysis!
People divided our data into seven groupings of academic study since there are so many distinctively nuanced - and suitably titled - degrees in the literary world:
- Statistics and mathematics: covers statistics and mathematics-centered degrees
- Data science and analysis: includes machine learning;
- Engineering: Natural sciences (physics, chemistry, and biology);
- Economics and social sciences: which comprise economics, finance, business, politics, psychology, philosophy, history, marketing, and management studies.
Data scientists in their first year of employment accounted for 13% of our 2020 data.
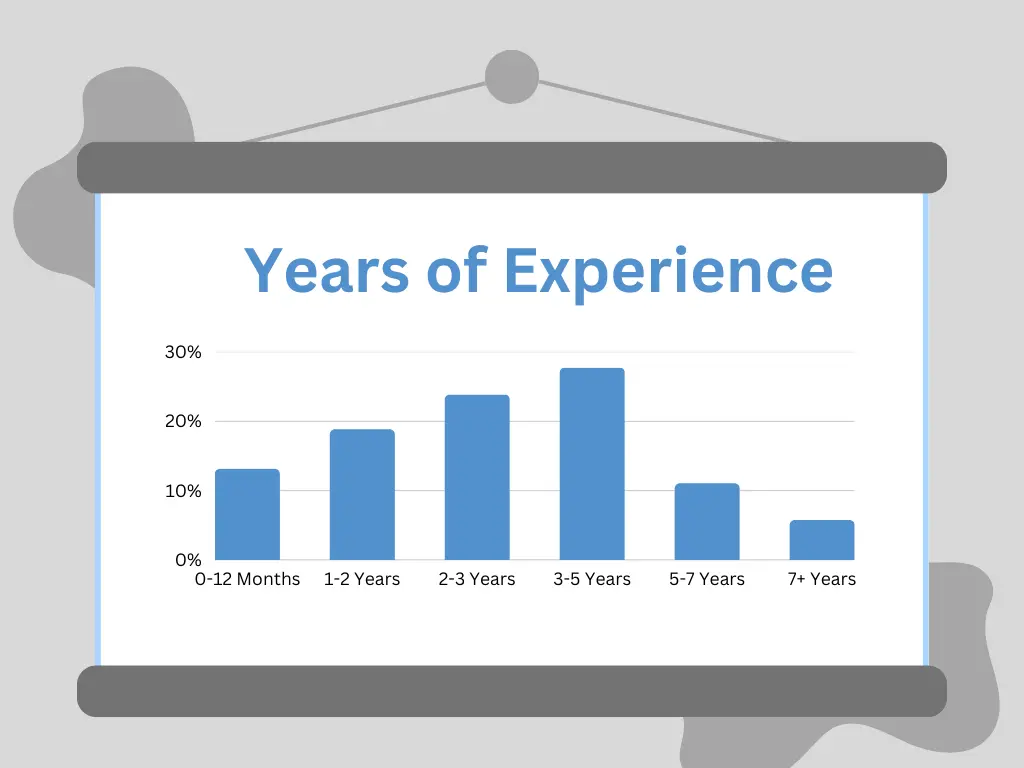
These are all fascinating numbers, especially when compared to data from 2019 and 2018. More precisely, we are seeing an almost 50% decline in the number of data scientists starting out in 2020 (13%), compared to data scientists starting out in 2019 and 2018 (25%). Given the growth in average data scientist experience, we may deduce that these experts remain in the industry, making it more difficult for junior individuals to enter.
Data Scientists Must Have Programming Skills
- The rivalry between Python and R in the data science community has been a topic of discussion.
- While Python has gained significant popularity and surpassed R in recent years, it is important to recognize the strengths and use cases of both languages.
- Python's rise in data science can be attributed to its versatility as a general-purpose programming language, while R has long been recognized as a language designed specifically for statistical analysis and data visualization.
- SQL plays a crucial role in data science as it provides a standardized language for working with relational databases.
- Data scientists often utilize a combination of these languages and tools to leverage their strengths and meet the diverse demands of their projects.
- As you are going to read that the opportunities for Data scientists are unmatchable as compared to other career opportunities, it is better to learn these skills at the earliest. If you are interested in a course, then consider some top rated Data science courses such as from Scaler which will cover all the mentioned skills and languages.

Industry Demanding Data Scientists
Technology Sector:
- Use data to improve the user experience, improve products, and drive innovation.
- Machine learning and AI approaches may be used in a variety of applications, including natural language processing, computer vision, and recommendation systems.
- Large datasets should be analyzed to get insights into user behavior and enhance company strategy.
- Create data mining, pattern recognition, and predictive modeling algorithms.
Banking and finance:
- To detect fraud, manage risk, and make data-driven investment decisions, use data analysis and modeling.
- Create credit scoring models, consumer segmentation, and tailored financial solutions.
- Machine learning algorithms can be used in algorithmic trading and portfolio optimization.
- Data can be used for regulatory compliance and reporting.
Pharmaceuticals and healthcare:
- Clinical data analysis is used to enhance patient outcomes, generate personalized treatment, and optimize healthcare operations.
- Machine learning can be used in illness diagnosis, medication discovery, and genomics research.
- Predictive modeling may be used to detect high-risk patients and optimize treatment options.
- Analyze and make decisions using electronic health records (EHR) and medical imaging data.
Retailing and e-commerce:
- Use consumer data to better understand purchasing habits, personalize suggestions, and optimize pricing tactics.
- For demand forecasting, inventory management, and supply chain optimization, employ data-driven methodologies.
- Understand the opinions of consumers and increase the reputation of a company by using sentiment analysis and social media mining.
- For marketing campaign optimization, use A/B testing and experimentation.
Telecommunications:
- Analyze client usage trends and behavior to enhance pricing strategies and customer happiness.
- Use machine learning to forecast churn and retain customers.
- Optimize network performance, capacity planning, and service quality by analyzing network data.
- For brand monitoring and consumer sentiment research, use sentiment analysis and social media mining.
Academia Opportunities
Research Possibilities
- Conduct cutting-edge data science research while investigating fresh approaches, formulas, and strategies.
- To benefit the academic community, publish research articles in renowned journals and conferences.
- Work together with other academics to solve complicated challenges and stimulate innovation, both inside academia and across industrial relationships.
- Obtain financing and research grants to assist with initiatives and promote data-driven innovations.
Mentoring and Teaching
- Teach undergraduate and graduate courses in order to instruct and motivate the upcoming generation of data scientists.
- Create educational materials on subjects like statistics, artificial intelligence, data mining, and data visualization.
- Students conducting data science research projects or theses should get mentoring and direction.
- Create an environment in the classroom that promotes analytical thinking, problem-solving, and the use of data science tools.
Entrepreneurship Opportunities
Economic Opportunities
- Discover areas where data science can provide value and provide solutions to challenging issues by investigating various sectors and disciplines.
- Analyse market trends and consumer demands to find any shortages or unmet needs that data-driven solutions may satisfy.
- In order to determine the viability and potential effect of your entrepreneurial enterprise, do market research and feasibility studies.
Create a Data-Driven Product or Service
- Create cutting-edge, data-driven goods or services that address particular market demands or current industry difficulties.
- Create intelligent, scalable solutions by using data science techniques like machine learning, predictive analytics, and natural language processing.
- Make sure that your product or service helps clients make better decisions or operate more efficiently by offering them practical information.
Investment and handling finances
- Find funding options from venture capitalists, angel investors, or government grants to assist in the initial investment and growth of your data science business.
- Make sure to have a comprehensive financial plan and effectively manage resources to ensure long-term sustainability and development.
- By monitoring key performance indicators, or KPIs, you can monitor the development and success of your business venture.
Partnerships and Collaborations:
- Work in collaboration with other companies, academic institutions, or start-ups to explore new market opportunities, benefit from synergies, and get access to complementary expertise.
- To extend your data science offerings and capabilities, create strategic relationships with business executives, IT service providers, and information suppliers.
- To find like-minded businessmen, mentors, and investors, join entrepreneurial networks, events, and incubators.
With a career in data science entrepreneurship, you can combine your love of innovation and data with the chance to launch a company that makes use of data-driven solutions. It gives you the freedom to follow your own vision, make a difference, and support the larger data science ecosystem.
Conclusion
- Numerous job options in business, academia, and industry are available in data science. Data scientists use their expertise in the workplace to influence corporate choices and create novel solutions.
- Opportunities for research, teaching, and advancing knowledge are provided by academia. Data scientists may launch their own businesses and develop data-driven goods and services through entrepreneurship. No matter the route taken, mastery in statistics, programming, and machine learning is crucial. Success requires constant learning, flexibility, and good communication.
- Data science provides exciting and profitable chances for professionals to make an effect and change the future because there is an ever-increasing need for data-driven insights.